
In addition to this external generalization issue, it is, in general, difficult for CNN-based image reconstruction networks to accurately reconstruct raw holograms of samples due to the limited receptive field of convolutional layers, which casts another challenge considering the relatively large scale of holographic diffraction patterns of samples. Some studies have explored using transfer learning to address this challenge, which requires fine-tuning the network using a subset of the new types of samples 48, 49, 50. On the other hand, “external generalization” to new objects from entirely new types of samples, never seen by the network before, remains a major challenge for deep neural networks, which might lead to image reconstruction degradation or hallucinations. Stated differently, this earlier body of work has successfully demonstrated the “internal generalization” of the hologram reconstruction and phase retrieval networks to new objects of the same sample type as used in training. In these earlier demonstrations, various deep network architectures, such as e.g., U-net-based convolutional neural networks (CNNs) 23, 25, 27, 33, 37, recurrent neural networks (RNNs) 42, 46, as well as generative adversarial networks (GANs) 31, 37, 42, 43, 47 have been proven to be effective for phase retrieval and hologram reconstruction for new (unseen) objects that belong to the same sample type of interest used during the training process. Some of the earlier results have also reported simultaneous performance of phase retrieval and autofocusing in a single network architecture, demonstrating holographic imaging over an extended depth-of-field 25, 34, 42. Recent work has also utilized deep neural networks 23, 24, 25, 26, 27, 28, 29, 30, 31, 32, 33, 34, 35, 36, 37, 38, 39, 40, 41, 42, 43, 44, 45 to reconstruct the complex sample field from a hologram in a single forward inference step, achieving an image reconstruction quality comparable to iterative hologram reconstruction algorithms that are based on physical wave propagation.


Due to the missing phase information, various computational approaches have been developed to digitally reconstruct holograms 13, 14, 15, 16, 17, 18, 19, 20, 21, 22.

Beyond holographic microscopy and quantitative phase imaging, FIN and the underlying neural network architecture might open up various new opportunities to design broadly generalizable deep learning models in computational imaging and machine vision fields.ĭigital holography provides unique advantages in microscopic imaging, by reconstructing the complex optical fields of input samples 1, 2, 3, 4, 5, 6, 7, 8, 9, 10, 11, 12. We experimentally validated the performance of FIN by training it using human lung tissue samples and blindly testing it on human prostate, salivary gland tissue and Pap smear samples, proving its superior external generalization and image reconstruction speed. Compared with existing convolutional deep neural networks used for hologram reconstruction, FIN exhibits superior generalization to new types of samples, while also being much faster in its image inference speed, completing the hologram reconstruction task in ~0.04 s per 1 mm 2 of the sample area. FIN architecture is based on spatial Fourier transform modules that process the spatial frequencies of its inputs using learnable filters and a global receptive field. Here we introduce a deep learning framework, termed Fourier Imager Network (FIN), that can perform end-to-end phase recovery and image reconstruction from raw holograms of new types of samples, exhibiting unprecedented success in external generalization. However, the generalization of their image reconstruction performance to new types of samples never seen by the network remains a challenge.
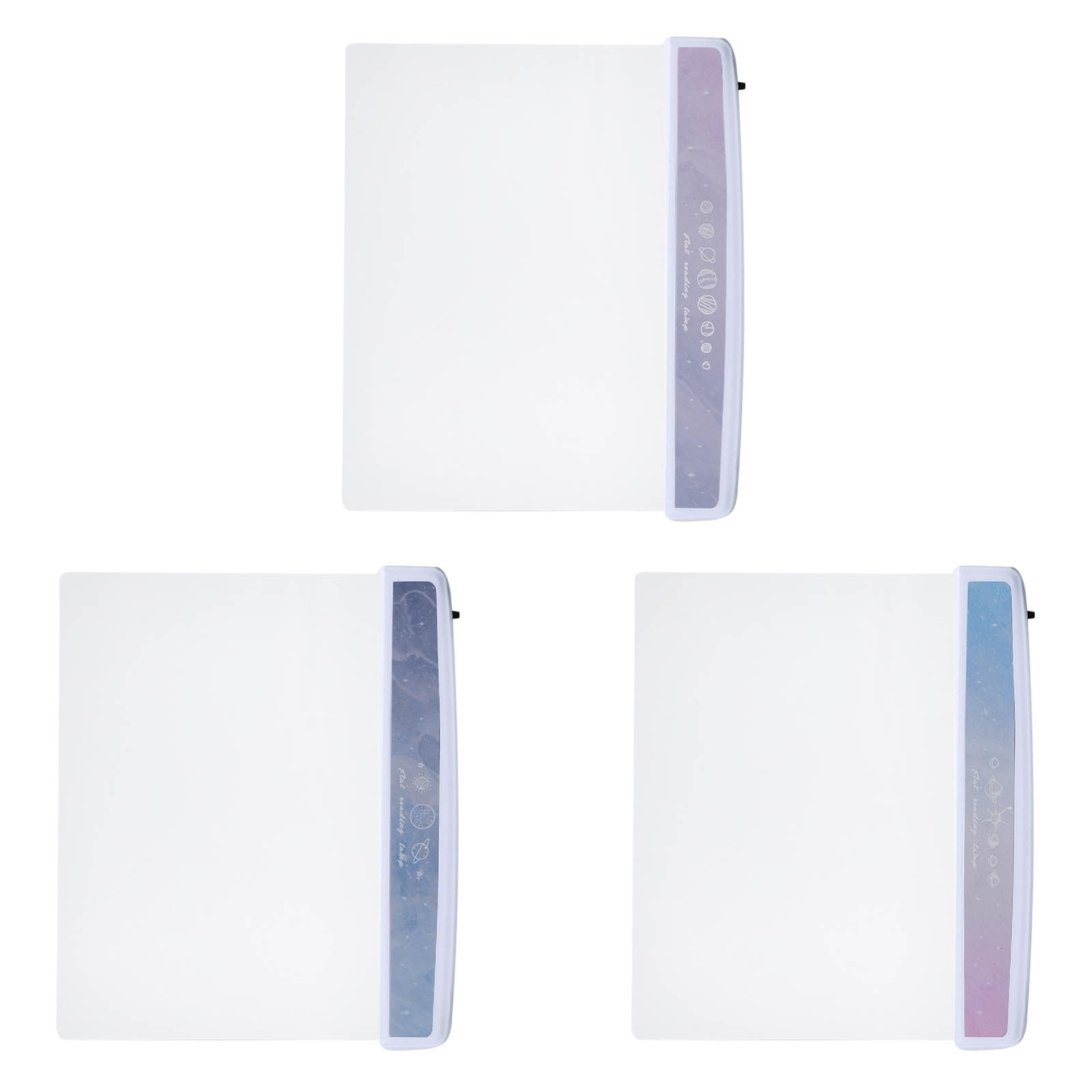
Deep learning-based image reconstruction methods have achieved remarkable success in phase recovery and holographic imaging.
